AI technologies can help combat disinformation
Leanne Manas is a household name in South African television. In late 2023, the morning news presenter’s face appeared in fake news stories and advertisements, where “she” seemingly promoted various products and get-rich-quick schemes. She had fallen victim to “deep faking”.
Meanwhile, in Hong Kong, a finance worker was tricked into paying out $25 million in a sophisticated scheme whereby fraudsters impersonated the company’s Chief Financial Officer and other colleagues in a video conference call using deepfake technology.
Deepfakes are media that have been digitally manipulated (typically using artificial intelligence) to replace one’s likeness. This does not require cutting-edge technical know-how; free tools like FaceSwap and Zao mean that anybody can create deepfakes.
Once, “seeing was believing”, but in today’s age of deepfakes and other AI-assisted fabrications, the foundations of trust and belief are being challenged as never before. The World Economic Forum identifies this and other forms of mis/disinformation as the biggest risk to global stability in the next two years, even more dangerous than interstate armed conflict, extreme weather, and inflation.
They predict that “…over the next two years, the widespread use of misinformation and disinformation, and tools to disseminate it, may undermine the legitimacy of newly elected governments. Resulting unrest could range from violent protests and hate crimes to civil confrontation and terrorism.”
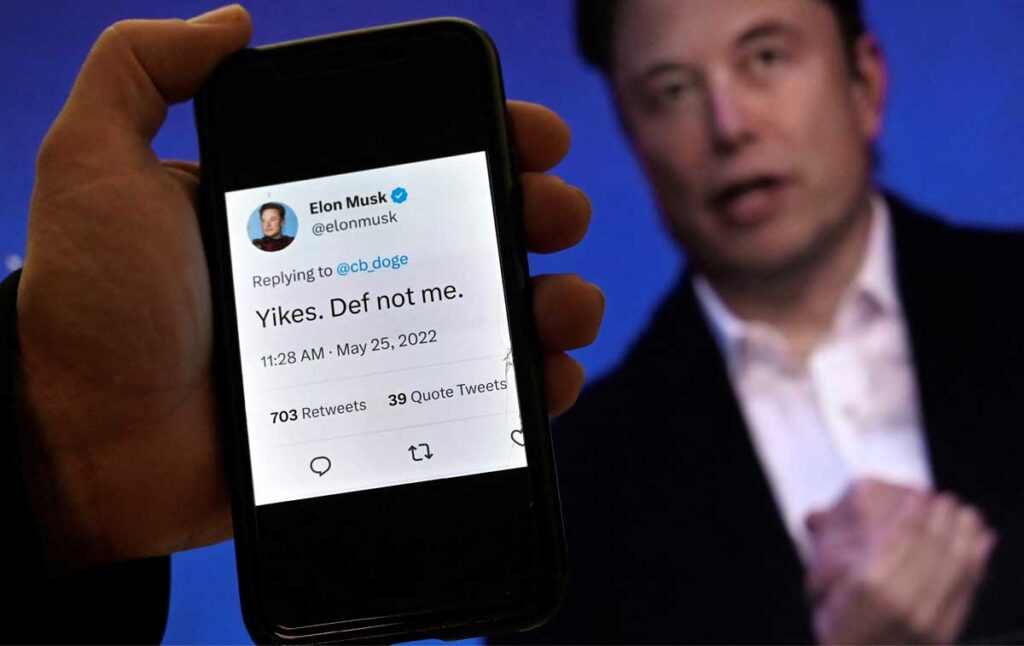
AI acts as a double-edged sword of disinformation. On the one hand, it fuels the development and spread of disinformation with frightening ease. Generative AI systems like GPTs and tools for creating deepfakes allow anyone to create and disseminate fake text, images, audio, and video. This, in turn, presents threats to our socio-political and economic systems.
However, AI can also be a powerful ally in detecting and mitigating fake content. As AI technology advances in sophistication, relying solely on human capabilities becomes inadequate for detecting mis/disinformation. One key field in AI-based mitigation is machine learning, which enables computers to learn from large data sets and improve their performance over time without explicit programming.
Machine learning techniques like network analysis, classifiers, sentiment and semantic analysis, anomaly detection, and pattern recognition can be used to identify patterns, institutions, and actors linked to disinformation in social and news media. They can also be trained to differentiate authentic media from AI-generated ones.
Disinformation campaigns rarely operate in isolation. Network analysis can combat disinformation by mapping the spread of false information and identifying key actors. Imagine a social media network where fake news spreads fast: by analysing the connections between accounts sharing the story, researchers can identify influential users or bots pushing the narrative. Similarly, in the news media, network analysis can be used to identify journalists, news outlets, and other actors associated with disinformation, especially given the recent rise in disinformation-spreading news outlets.
Machine learning classifiers, trained on large datasets of labelled information, can also be used to identify patterns and differentiate fake and authentic content using features extracted from the data.
In a recent working paper from the Brookings Institution, a think-tank based in Washington, Maryam Saeedi, an economist at Carnegie Mellon University, and her colleagues combined these two techniques to analyse 62 million posts from X (formerly Twitter) written in Farsi.
Their focus was on the wave of anti-government protests in Iran that began in September 2022, with specific interest in “spreader accounts”, which they dubbed “imposters”. These accounts initially posed as allies of the protesters but later shifted to disseminating disinformation to undermine the protests.
Initially, the researchers manually identified several hundred imposter accounts. Subsequently, they developed a machine learning classifier trained to detect more imposters with similar traits, including their posting behaviour, follower patterns, hashtag usage, account creation dates, and other metrics. The researchers managed to replicate the identification of these imposter accounts with 94% accuracy using network analysis alone without exploring the content of their posts.
According to the researchers, their methodology’s advantage is that it identifies “accounts with a high propensity to engage in disinformation campaigns, even before they do so.” They propose that social media platforms could use these network analysis methods to compute a “propaganda score” for each account, visible to users, to indicate the likelihood that an account engages in disinformation.
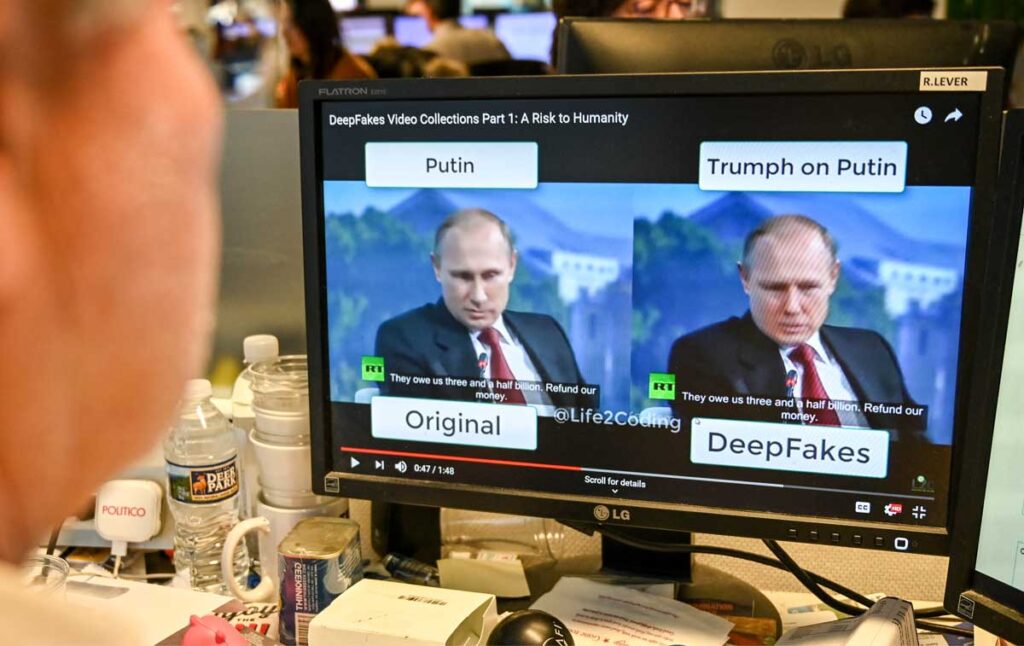
Semantic and sentiment analysis are also important in fighting disinformation. When many accounts use the same wording, spotting disinformation is relatively simple. But the language of disinformation is often manipulative – it may take different shapes or use different wording to push the same narrative. Semantic techniques like embeddings can address this by converting text into numerical representations that capture meaning based on their contexts of use. The algorithm processes millions of documents, sentences, and word tokens, learning the meanings of text from the company they keep. Words, sentences, and larger text segments with similar semantic meanings are positioned close together in this vector space.
By using embeddings, sentiment analysis can, for example, go beyond simple keyword and dictionary-based matching to understand the context and nuances of disinformation language, which often aims to evoke strong emotional reactions such as fear, anger, or distrust.
Valent, a US-based social media threat monitoring organisation, uses similar techniques to fight disinformation. Its AI tool, Ariadne, takes in feeds from social platforms and looks for common underlying narratives and sentiments to spot unusual, coordinated action. This method, according to the organisation, outperforms keyword-based approaches used for sentiment analysis.
Media forensics are also essential in combating disinformation. Disinformation often relies on manipulating images and videos to bolster false narratives. Reverse image search algorithms, a staple of AI-powered search engines, can expose these forgeries. By comparing a suspicious image across vast online databases, AI can determine if it has been tampered with or originates from an unrelated event.
AI behavioural and physiological analysis techniques are also part of media forensics and can make a difference. This examines specific behaviours and physical characteristics of individuals in media content to verify its authenticity.
DARPA, the special projects research arm of the US Department of Defense, has been investing in the “semantic forensics” (SemaFor) programme to detect, attribute and characterise manipulated and synthesised media as well as creating a toolbox of defences.
For example, to differentiate between the authentic and fake video of a government official, the tool can identify the inconsistencies and errors that are often omitted in automated manipulation. By analysing for body inconsistencies like mismatched lip movements and earrings in videos or illogical object placement in images, such techniques can expose forgeries even when the technical manipulation itself is flawless.
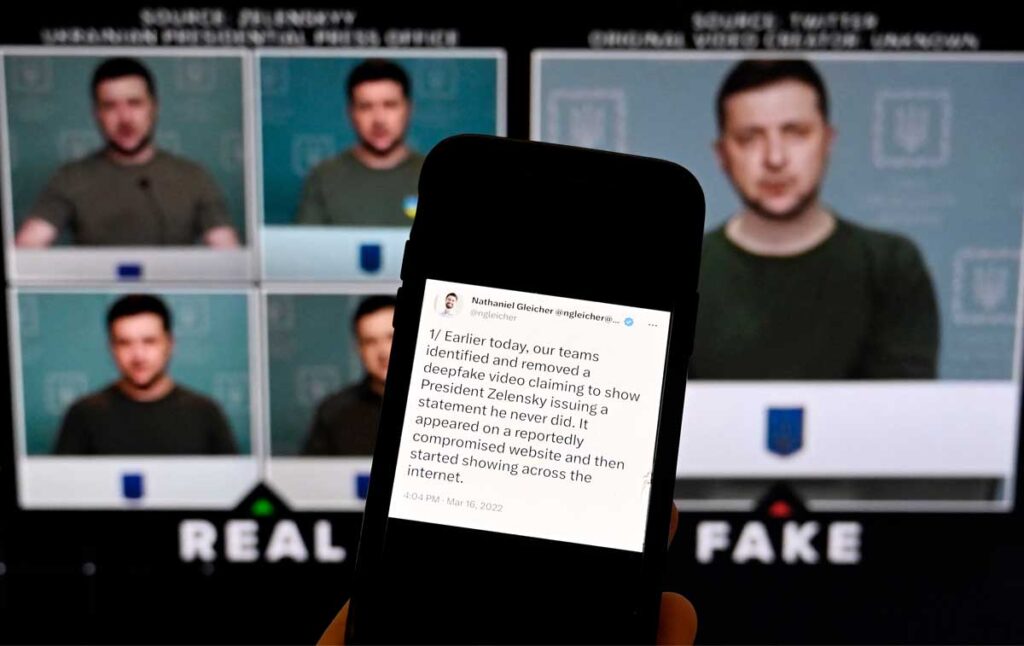
Using authentic data of the person in question, it is also possible to train a model that learns their characteristics, such as patterns of head tilt or facial movements while speaking. This can be combined with other techniques, such as heartbeat detection from video, which is difficult to fake and can be spotted by looking for tiny variations in skin colour, particularly on the forehead. This “asymmetric advantage” can be used to identify one inconsistency, which can expose the entire fabrication, says DARPA.
Despite AI’s immense potential, human expertise remains vital. Crowdsourced verification platforms employ the power of the public to verify information and identify disinformation. AI can pre-screen user content and flag potential instances of disinformation for human fact-checkers to review and act. This technique combines the best of both worlds: the speed and large-scale processing capability of AI with the critical thinking and judgment of experienced fact-checkers for complex tasks (like language understanding and ensuring fairness and applicability in the system). Tech giant Meta uses this technique on all its platforms to fight misinformation.
While AI offers a powerful weapon against disinformation, challenges remain. One major challenge is that training data bias can lead AI tools to perpetuate existing prejudices. For example, in a forthcoming article, researchers Nnaemeka Ohamadike, Kevin Durrheim, and Mpho Primus found that neural word embeddings trained on South African COVID-19 vaccination news data from 2020 to 2023 learned a wide range of race-based socioeconomic and health biases (e.g., that whites are rich and taxpayers and blacks are social welfare reliant). Such biases reinforce harmful stigma, discrimination, and inequality, especially against marginalised groups. AI tools can reproduce and amplify these biases when used in downstream tasks like disinformation detection.
Detection tools can also make mistakes, flagging real content as fake and vice versa. For example, OpenAI, the creators of ChatGPT, admitted that even their detection tool had a poor 26% success rate in identifying AI-generated text and recommended combining it with other techniques for better results.
Despite challenges, AI technologies remain vital in this fight. Although no single technique can detect all disinformation, combining several of them can greatly improve accuracy. Detection tools will also need to constantly evolve, considering the fast-changing nature of disinformation.
Renée DiResta, the research manager of the Stanford Internet Observatory, is less convinced about the potency of today’s detection tools. While these tools might excel in controlled environments with ample analysis time, she argues they are ill-suited for real-time situations where snap judgments are crucial.
DiResta highlights a deeper concern: even flawless detection of deceptive media does not guarantee everyone will believe the truth. She points to the example of manipulated audio that swung a Slovakian election in 2023 in which a politician (who later lost the election) was heard discussing election rigging with a journalist. People can be resistant to fact-checks, DiResta says, especially from sources they distrust. This means solutions beyond just technology are needed to combat disinformation.
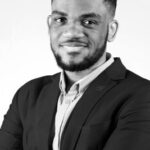
Nnaemeka is a Senior Data Analyst at Good Governance Africa. He holds a Master’s degree in e-Science (Data Science) from the University of the Witwatersrand, funded by South Africa’s Department of Science and Innovation. Much of his research explores socio-political issues like human development, governance, bias, and disinformation, using data science. He has published research in scholarly journals like EPJ Data Science, Politeia, the Journal of Social Development in Africa, and The Africa Governance Papers. He has experience working as a Data Consultant at DataEQ Consulting. He has also taught at the Federal University, Lafia (Nigeria) and the University of the Witwatersrand, Johannesburg (South Africa).